Researchers use digital cameras, machine learning to predict neurological disease
[Oct 17, 2022: Diana Yates, University of Illinois at Urbana-Champaign]
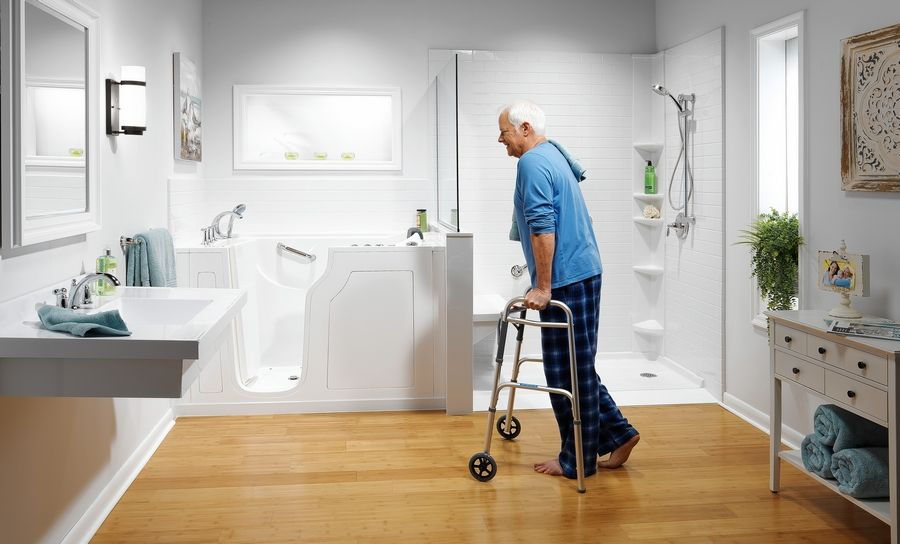
The goal of the research was to make the process of diagnosing these diseases more accessible. (CREDIT: Creative Commons)
In an effort to streamline the process of diagnosing patients with multiple sclerosis and Parkinson’s disease, researchers used digital cameras to capture changes in gait – a symptom of these diseases – and developed a machine-learning algorithm that can differentiate those with MS and PD from people without those neurological conditions.
Their findings are reported in the IEEE Journal of Biomedical and Health Informatics.
The goal of the research was to make the process of diagnosing these diseases more accessible, said Manuel Hernandez, a University of Illinois Urbana-Champaign professor of kinesiology and community health who led the work with graduate student Rachneet Kaur and industrial and enterprise systems engineering and mathematics professor Richard Sowers.
Currently, patients must wait – sometimes for years – to get an appointment with a neurologist to make a diagnosis, Hernandez said. And people in rural communities often must travel long distances to a facility where their condition can be assessed. To be able to gather gait information using nothing more than a digital camera and have that data assessed online could allow clinicians to do a quick screening that sends to a specialist only those deemed likely to have a neurological condition.
Related Stories
To conduct the study, the team videotaped adults with and without MS or Parkinson’s disease as they walked on a treadmill, focusing the digital cameras on participants’ hips and lower limbs. Those without the neurological conditions were age-, weight- and gender-matched with participants with MS and PD.
The walking exercise also included trials in which participants walked while reciting every-other letter of the alphabet in sequence. This added task was designed to mimic the real-world challenges of walking while engaging in other potentially mentally distracting tasks, Sowers said.
“This is a novel study in that we were trying to address the fact that the lab is different from how people behave in the wild,” he said. “When you’re at home, you’re doing whatever you’re doing, but you’re also thinking, ‘Did I close the garage door? Did I turn the stove off?’ So there’s an added cognitive load.”
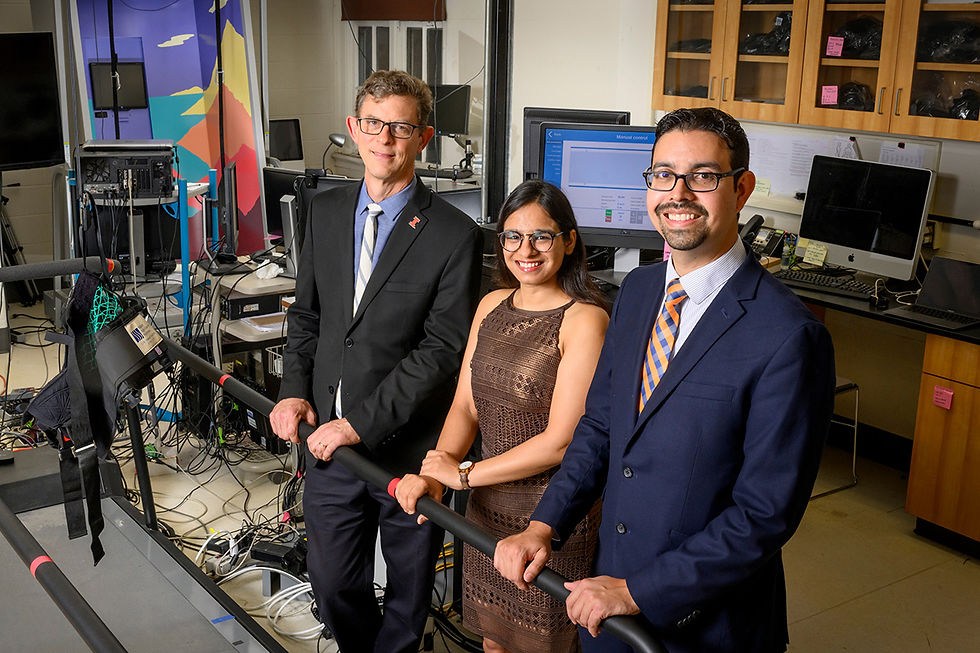
From left, Richard Sowers, Rachneet Kaur and Manuel Hernandez led the development of a new approach for identifying people with multiple sclerosis or Parkinson’s disease. Their method involves videotaping the hips and lower extremities of individuals walking on a treadmill and allowing a machine-learning algorithm to differentiate gait abnormalities associated with each of these neurological conditions. (CREDIT: Fred Zwicky)
The researchers used an open-source tool to analyze the video to extract data about how participants moved during the walking exercises.
“We looked at the body coordinates for hips, knees, ankles, the big and small toes and the heels,” said Kaur, who developed the method for analyzing how these coordinates moved over time to look for differences between adults with and without MS or Parkinson’s disease.

Base of Support and it's centroid throughout a stride starting at HSL. Subject's right (pink) and left (grey) feet keypoints, namely, little toe, big toe, and heel, are labelled (in order) as 0, 1, 2 and 3, 4, 5, respectively. The overground view of feet is shown for each support phase, where feet not in contact with ground are colored white. The green shaded regions, i.e. hexagon for the DS and triangle for the SS, define the BoS and the red x mark is it's centroid. (CREDIT: IEEE Xplore)
She tested the accuracy of her approach using more than a dozen traditional machine-learning and deep-learning algorithms. The team also tested the method on new study subjects to see if it could identify those with MS, those with Parkinson’s disease and those with neither condition.
The study revealed that several of the algorithms were more than 75% accurate at detecting these differences.
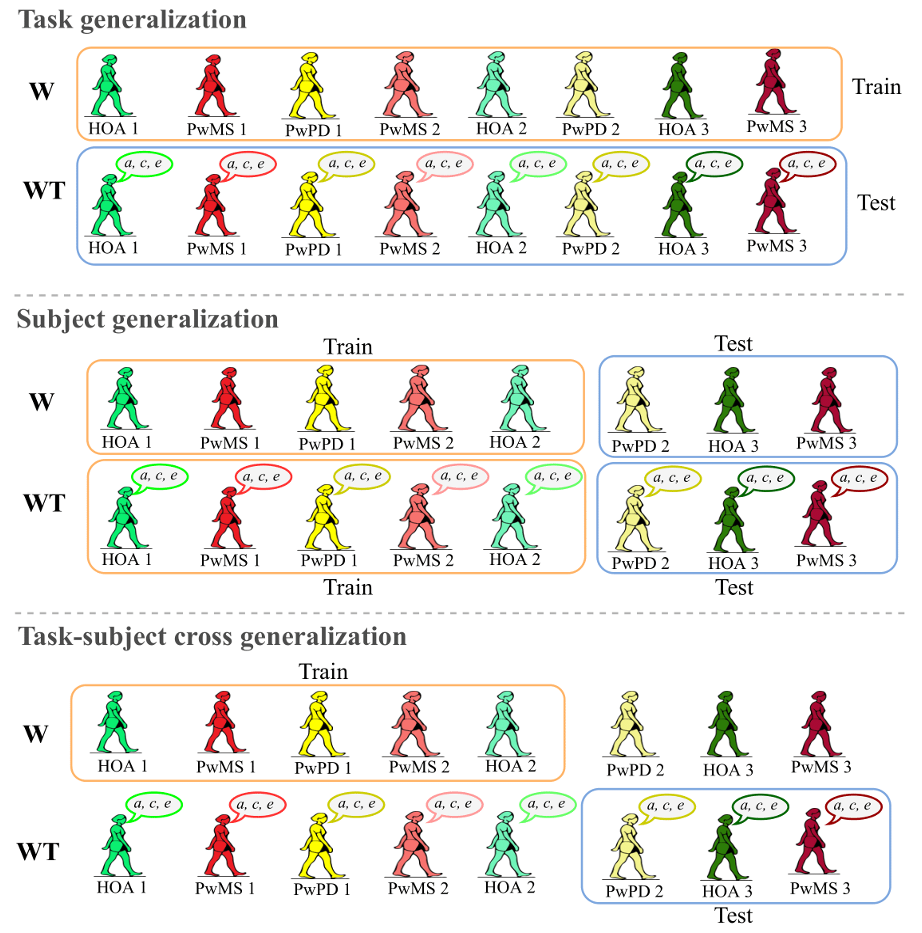
Classification frameworks. Top: Task-gen, Middle: Subject-gen, Bottom: Cross-gen. HOA, PwMS and PwPD are depicted in shades of green, red and yellow, respectively. The indices (1, 2, 3, ...) are used as a reference for dummy subject identifiers. (CREDIT: IEEE Xplore)
“This study suggests the viability of inexpensive vision-based systems for diagnosing certain neurological disorders,” the researchers wrote.
Making the new tools available to the public will likely take several years, the scientists said.
Hernandez and Sowers are faculty in the Carle Illinois College of Medicine at Illinois. Hernandez also is affiliated with the Beckman Institute for Advanced Science and Technology at the U. of I. Sowers also is affiliated with the U. of I. National Center for Supercomputing Applications.
The paper “A vision-based framework for predicting multiple sclerosis and Parkinson’s disease gait dysfunctions – a deep learning approach” is available online and from the U. of I. News Bureau.
For more science news stories check out our New Discoveries section at The Brighter Side of News.
Note: Materials provided above by University of Illinois at Urbana-Champaign. Content may be edited for style and length.
Like these kind of feel good stories? Get the Brighter Side of News' newsletter.
Comments